Loading...
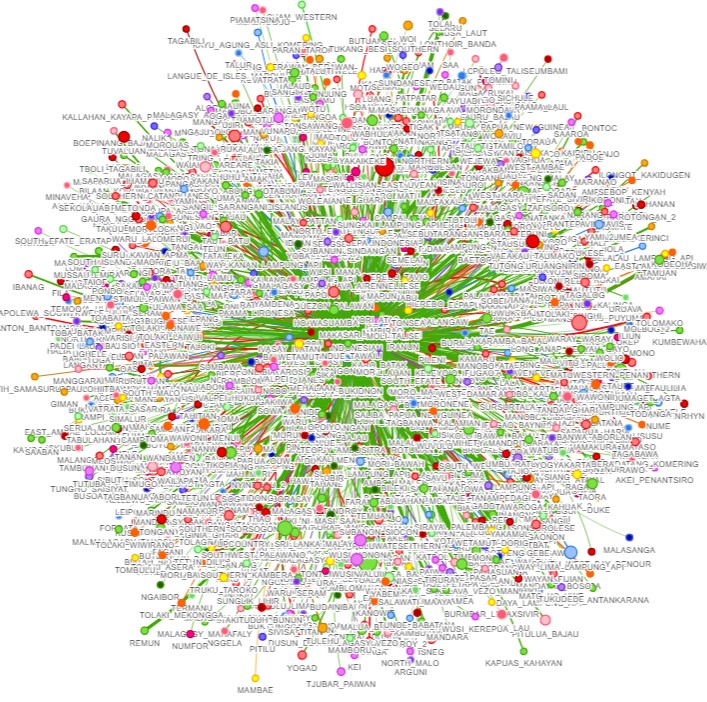
Indonesia Language Sphere
- There are more than 7000 languages around the world. However, 95 % of the world population speak only 5 % of them, at most 400 languages. More than half of them have fewer than 10,000 speakers. In 2010, UNESCO released a list of 2,464 endangered languages. In Indonesia, 144 languages are endangered. To preserve and increase the use of those languages, Professor Yohei Murakami and team started the Indonesia Language Sphere project. The purpose of this project is to develop comprehensive sets of bilingual dictionaries for Indonesian ethnic languages. To this end, a generalized bilingual lexicon induction method that combines pairs of existing dictionaries has been proposed. Furthermore, to reduce the total cost of bilingual dictionary creation, the machine and manual creation processes were combined and a planner that optimizes creation orders were constructed. Following paper introduces the proposed methods and reports a preliminary experiment result focusing on Indonesian, Malay, Javanese, Sundanese, and Minangkabau.
- Publication:
- Murakami, Y., 2019, March. Indonesia Language Sphere: an ecosystem for dictionary development for low-resource languages. In Journal of Physics: Conference Series (Vol. 1192, No. 1, p. 012001). IOP Publishing. [full paper]
Statistics of Total Bilingual Dictionaries
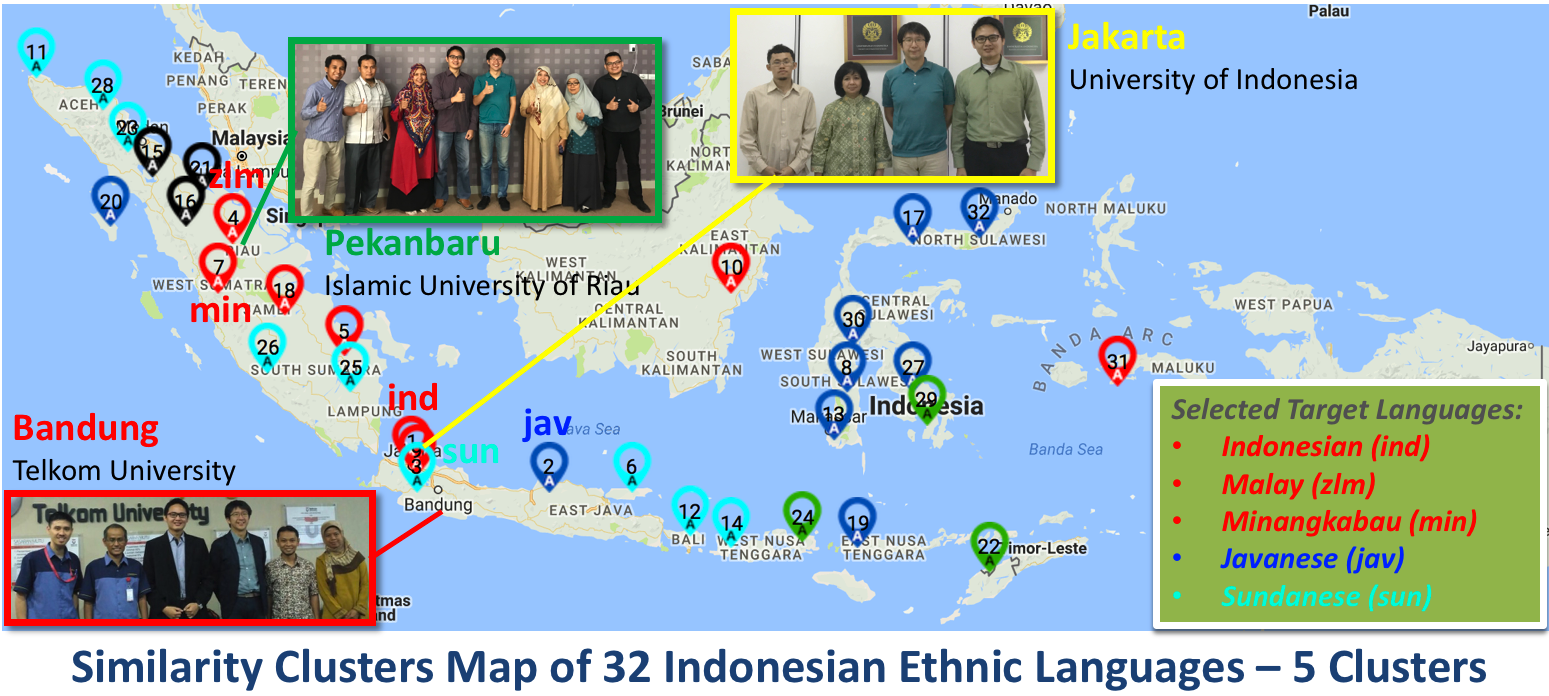
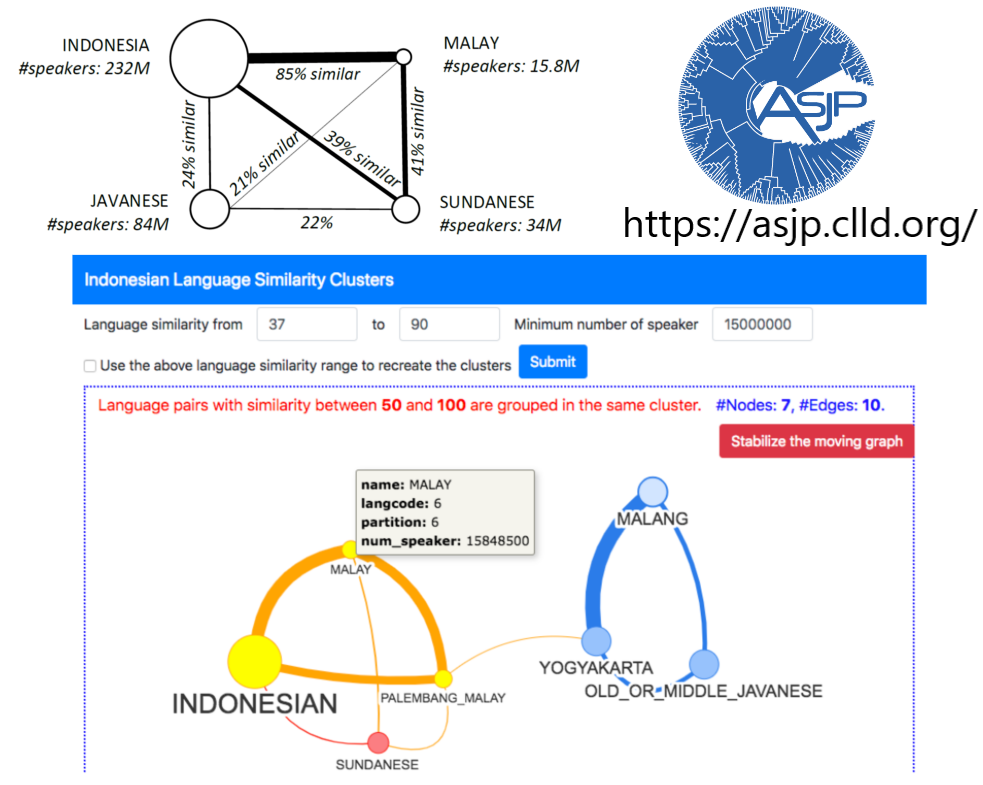
Language Similarity Clustering
- Lexicostatistic and language similarity clusters are useful for computational linguistic researches that depends on language similarity or cognate recognition. Nevertheless, there are no published lexicostatistic/language similarity cluster of Indonesian ethnic languages available. We formulate an approach of creating language similarity clusters by utilizing ASJP database to generate the language similarity matrix, then generate the hierarchical clusters with complete linkage and mean linkage clustering, and further extract two stable clusters with high language similarities. We introduced an extended k-means clustering semi-supervised learning to evaluate the stability level of the hierarchical stable clusters being grouped together despite of changing the number of cluster. The higher the number of the trial, the more likely we can distinctly find the two hierarchical stable clusters in the generated k-clusters. However, for all five experiments, the stability level of the two hierarchical stable clusters is the highest on 5 clusters. Therefore, we take the 5 clusters as the best clusters of Indonesian ethnic languages. Finally, we plot the generated 5 clusters to a geographical map.
- Publication:
- Nasution, A.H., Murakami, Y. and Ishida, T., 2019. Generating similarity cluster of Indonesian languages with semi-supervised clustering. International Journal of Electrical and Computer Engineering (IJECE), 9(1), pp.1-8. [full paper]
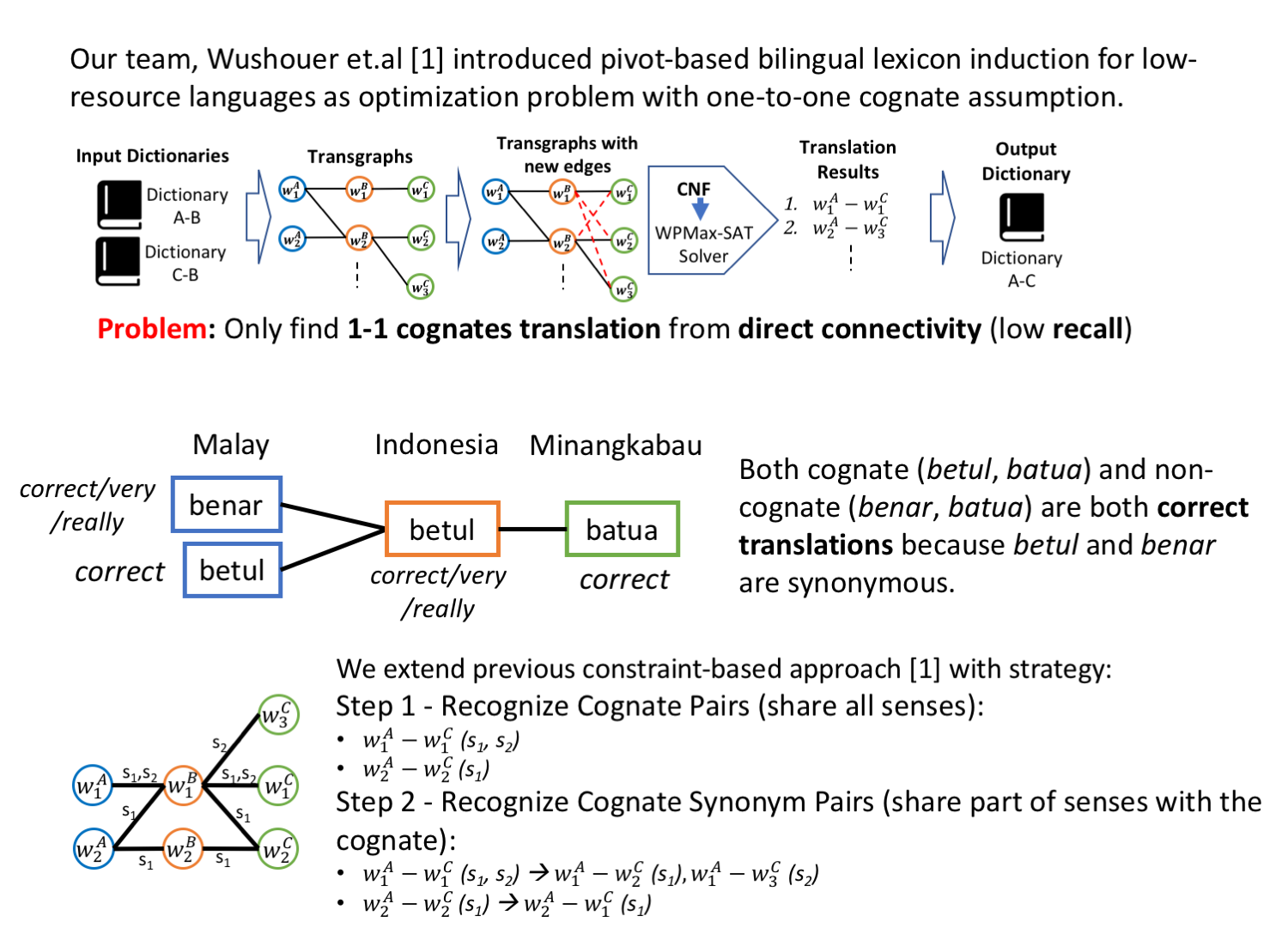
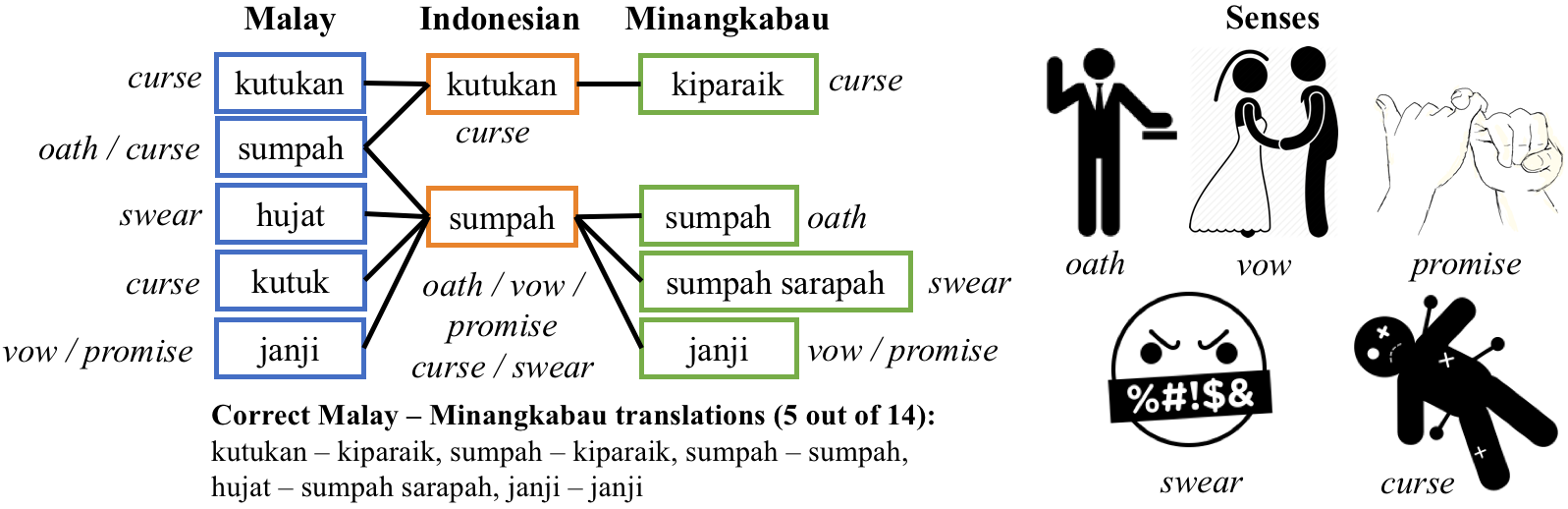
Bilingual Dictionary Induction
- The lack or absence of parallel and comparable corpora makes bilingual lexicon extraction a difficult task for low-resource languages. The pivot language and cognate recognition approaches have been proven useful for inducing bilingual lexicons for such languages. We propose constraint-based bilingual lexicon induction for closely-related languages by extending constraints from the recent pivot-based induction technique and further enabling multiple symmetry assumption cycle to reach many more cognates in the transgraph. We further identify cognate synonyms to obtain many-to-many translation pairs. This paper utilizes four datasets: one Austronesian low-resource language and three Indo-European high-resource languages. We use three constraint-based methods from our previous work, the Inverse Consultation method and translation pairs generated from Cartesian product of input dictionaries as baselines. We evaluate our result using the metrics of precision, recall and F-score. Our customizable approach allows the user to conduct cross validation to predict the optimal hyperparameters (cognate threshold and cognate synonym threshold) with various combination of heuristics and number of symmetry assumption cycles to gain the highest F-score. Our proposed methods have statistically significant improvement of precision and F-score compared to our previous constraint-based methods. The results show that our method demonstrates the potential to complement other bilingual dictionary creation methods like word alignment models using parallel corpora for high-resource languages while well handling low-resource languages. In 2022, we proposed to create a bilingual dictionary between ethnic languages using a neural network approach to extract transformation rules using character level embedding and the Bi-LSTM method in a sequence-to-sequence model. The model has an encoder and decoder. The encoder functions read the input sequence, character by character, generate context, then extract a summary of the input. The decoder will produce an output sequence where every character in each time-step and the next character that comes out are affected by the previous character. The current case for experiment translation focuses on Minangkabau and Indonesian languages with 13761-word pairs. For evaluating the model’s performance, 5-Fold Cross-Validation is used.
- Publications:
- Resiandi, K., Murakami, Y. and Nasution, A.H., 2022, June. A Neural Network Approach to Create Minangkabau-Indonesia Bilingual Dictionary. In Proceedings of the 1st Annual Meeting of the ELRA/ISCA Special Interest Group on Under-Resourced Languages (pp. 122-128). [full paper]
- Nasution, A.H., Murakami, Y. and Ishida, T., 2017. A generalized constraint approach to bilingual dictionary induction for low-resource language families. ACM Transactions on Asian and Low-Resource Language Information Processing (TALLIP), 17(2), pp.1-29. DOI: https://doi.org/10.1145/3138815 [full paper]
- Nasution, A.H., Murakami, Y. and Ishida, T., 2016, May. Constraint-based bilingual lexicon induction for closely related languages. In Proceedings of the Tenth International Conference on Language Resources and Evaluation (LREC'16) (pp. 3291-3298). [full paper]
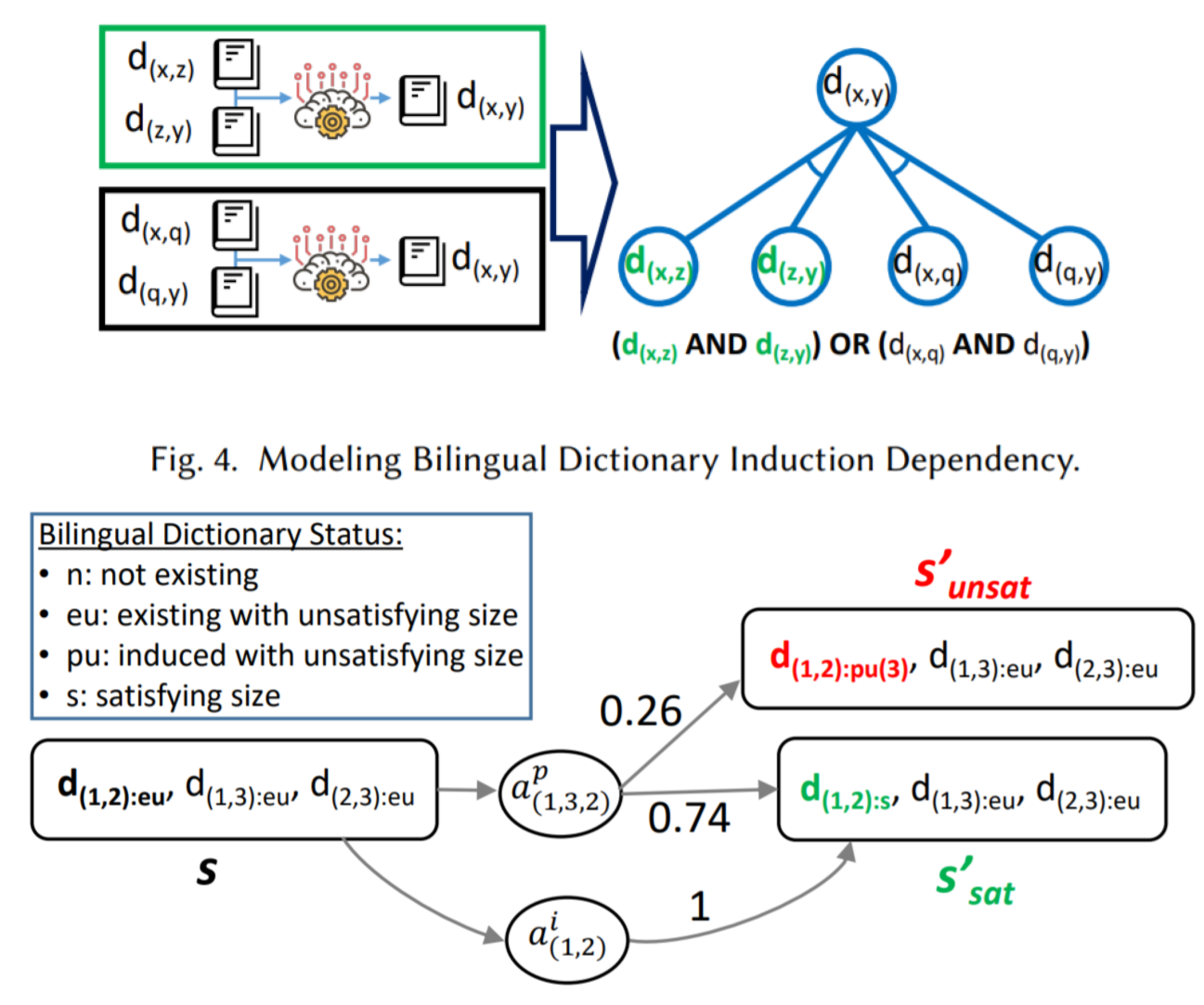
MDP Plan Optimizer
- The constraint-based approach has been proven useful for inducing bilingual lexicons for closely-related low resource languages. When we want to create multiple bilingual dictionaries linking several languages, we need to consider manual creation by bilingual language experts if there are no available machine-readable dictionaries are available as input. To overcome the difficulty in planning the creation of bilingual dictionaries, the consideration of various methods and costs, plan optimization is essential. We adopt the Markov Decision Process (MDP) in formalizing plan optimization for creating bilingual dictionaries; the goal is to better predict the most feasible optimal plan with the least total cost before fully implementing the constraint-based bilingual dictionary induction framework.
- Publication:
- Nasution, A.H., Murakami, Y. and Ishida, T., 2021. Plan optimization to bilingual dictionary induction for low-resource language families. Transactions on Asian and Low-Resource Language Information Processing, 20(2), pp.1-28. [full paper]
- Nasution, A.H., Murakami, Y. and Ishida, T., 2018, May. Designing a collaborative process to create bilingual dictionaries of Indonesian ethnic languages. In Proceedings of the Eleventh International Conference on Language Resources and Evaluation (LREC 2018). [full paper]
- Nasution, A.H., Murakami, Y. and Ishida, T., 2017, September. Plan optimization for creating bilingual dictionaries of low-resource languages. In 2017 International Conference on Culture and Computing (Culture and Computing) (pp. 35-41). IEEE. DOI: https://doi.org/10.1109/Culture.and.Computing.2017.21
Team
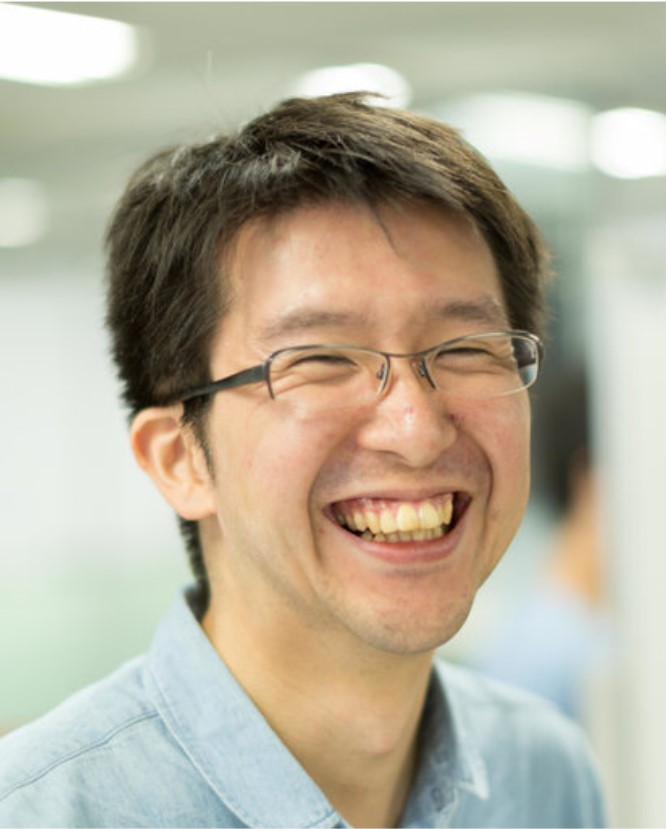
Associate Professor
College of Information Science and Engineering, Ritsumeikan University, Japan
Yohei Murakami
Ritsumeikan University, Japan
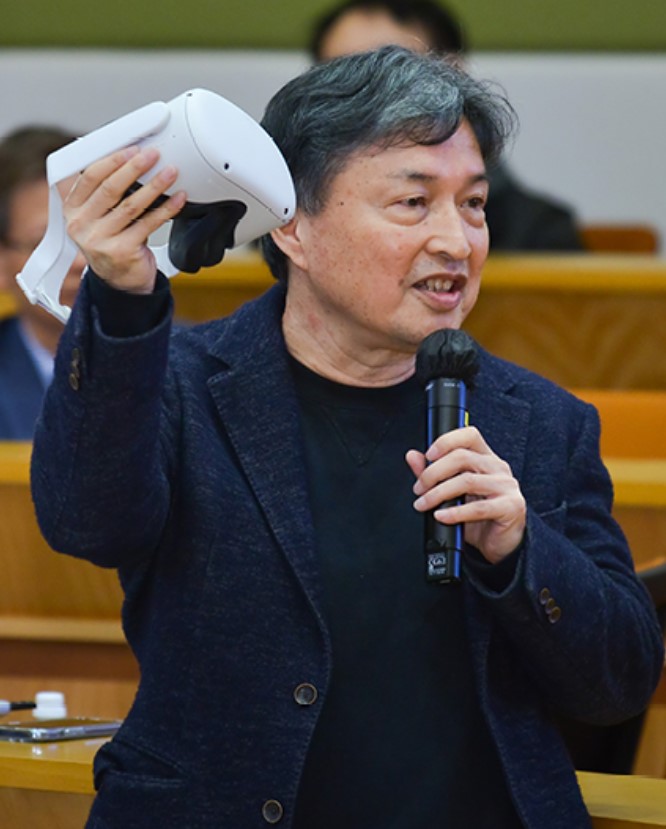
Professor Emeritus
Department of Social Informatics, Kyoto University, Japan
Toru Ishida
Kyoto University, Japan
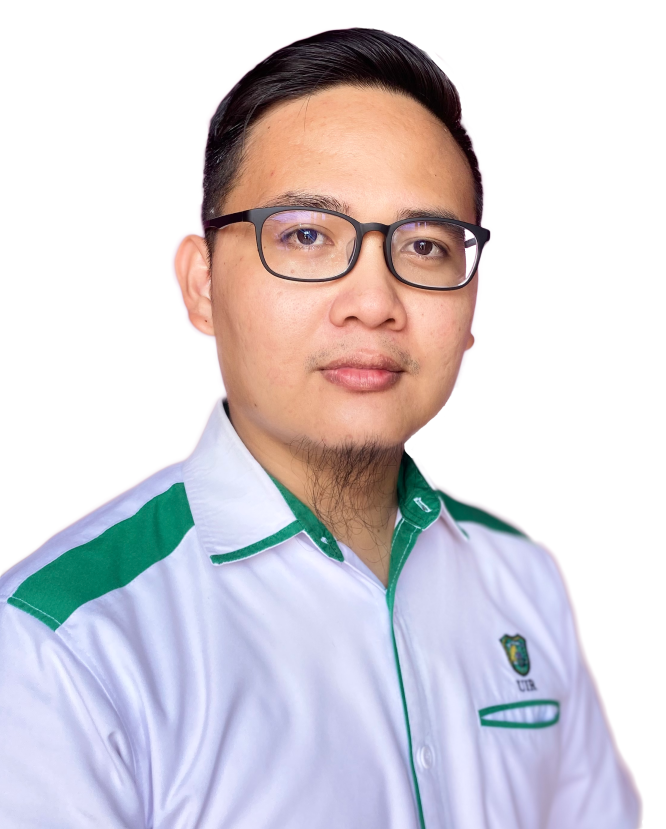
Associate Professor
Department of Informatics Engineering, Universitas Islam Riau, Indonesia
Arbi Haza Nasution
Universitas Islam Riau, Indonesia
Contact us
Project Leader
Professor Yohei MurakamiFaculty of Information Science and Engineering
Ritsumeikan University
E-mail: yohei@fc.ritsumei.ac.jp
URL: http://www.ritsumei.ac.jp/~yohei
Tel: (+81)-77-561-5065